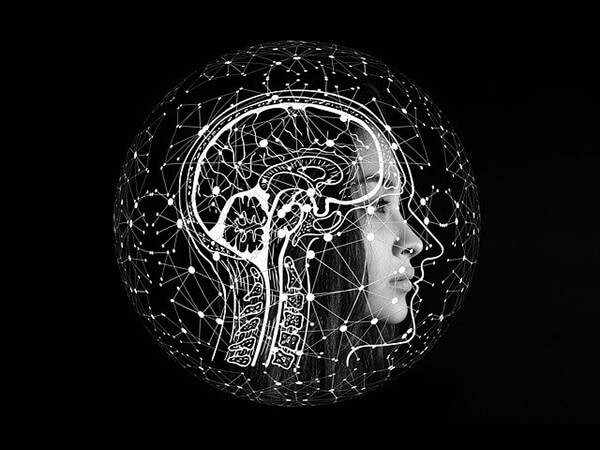
Otis Robinson explores the past, present and future of artificial intelligence (AI) in manufacturing, the barriers blocking adoption and commoditisation, and the application of AI in the garment manufacturing sector.
1. Upgrading manufacturing
Presently, global manufacturing industries need energising: quality, efficiency, cost and response times are primary considerations that require a boost [1, p. 13]. Worldwide consumption trends like fast fashion and customisation have piled extra pressures on enterprises, too, but digital technologies have helped some manufacturers to keep up with demand while keeping costs low, responses quick and quality high.
These tools have reduced the amount of brain-work humans have to manage in industrial settings. Manufacturing has high requirements that involve information sensing, analysis, decision-making, and operational control [1, p. 14] – requirements that are better suited to computational power – yet industries remain rooted in traditional practices.
It is suggested that new generation technologies like artificial intelligence (AI) could provide “historical opportunities for the revolutionary upgrading of the manufacturing industry into a powerful engine” capable of boosting economic and social development [1, p. 13]. Inspired by its potential, academics Ji et al even propose the upheaval of traditional manufacturing towards ‘new-generation intelligent manufacturing’ [1, p. 11]. This future model “represents an in-depth integration of new-generation [AI] technology and advanced manufacturing technology” [1, p. 11-12].
Nevertheless, even still, adoption of these technologies is somewhat at a stalemate, and according to academia, the continual development of the manufacturing industry “faces huge bottlenecks and difficulties” that urgently require the promotion of technological innovations and intelligent upgrades [1, p. 13].
2. About AI
Perhaps this stalemate is because, in many industries, AI is a phrase that conjures debate. People warn that AI could make human labour and human intelligence obsolete in the future, which encourages fearful and apocalyptic visions. On the contrary, the use of AI in an industrial setting is notably unlike this.
Dr John Oyekan, lecturer in digital manufacturing in the Department of Automatic Control & Systems Engineering at the University of Sheffield, UK, suggests this debate around AI is reductive.
He says: “If AI is used in the right way it will augment humans – make them more effective at their jobs and improve the productivity of the UK. That’s what we want as a nation.”
In fact, textile and garment manufacturing use cases are certain that AI is not the dystopian trigger many perceive it to be, and increasingly, evidence shows that industrial AI can indeed successfully create skilled job roles, increase customer value, improve productivity (for both operations and staff) and help discover key insights.
As an umbrella term, AI is a type of machine intelligence that acts autonomously and can make judgements and decisions based on specific goals or stimuli.
“AI works by combining large amounts of data with fast, iterative processing and intelligent algorithms, allowing the software to learn automatically from patterns or features in the data,” says analytics company SAS [2].
Despite fear orbiting the technology, people use it every day: mobile assistants Siri, Alexa and Cortana are a few modern examples of AI for personal use, though AI has a much wider scope and has been in development for decades.
Early research into AI began to explore computational problem solving in the 1950s; a decade later, the US Department of Defense “began training computers to mimic basic human reasoning”. This work is said to have ‘paved the way’ for the automation, formal reasoning, smart search and decision support systems visible in computing today [2].
Though the technology is associated with the mimicry of human intelligence and behaviour, its intelligence is limited only to the task it was programmed to do – this is known as ‘narrow intelligence’. It is well-suited to manufacturing settings and is unlike the ‘general intelligence’ visible in humans.
For example, narrow intelligence exists in the garment industry as fibre identification, predictive maintenance, quality control, improved computer-aided design (CAD) software and retail forecasting, among other applications.
However, some predict that AI technology may one day transition from ‘narrow AI’ to ‘general AI’ as the technology develops.
Greg Faughnan, sales director at EC Electronics, a manufacturing services company, elaborates: “The [AI] present in current technologies is seen as ‘narrow’ or ‘weak’ AI; it is designed to perform a specific task. […] However, the long-term objective of many developers is to create ‘general’ or ‘strong’ AI. Although narrow AI might be able to outperform humans at its specific task – be it solving equations or detecting cyber threats – strong AI [could] overtake humans at nearly every cognitive task.”
But unlike this frontier research discipline, industrial AI is “more concerned with the application of such technologies to address industrial pain-points for customer value creation, productivity improvement and insight discovery” [3].
Regardless, it is perhaps because of these complexities – and general misinformation about AI concepts – that many textile and garment industry businesses remain questionable of the technology, instead opting for entry-level digitalisation concepts like the Internet of Things (IoT); most companies ‘with heavy assets’ remain unaware of advancements in analytics and decision-support solutions offered by AI [4].
Although plenty of companies have adopted IoT tech, Jamie Hall, senior solutions specialist for Microsoft, says this alone is not enough. In an interview with The Manufacturer, Hall says companies need to combine the data acquired from connected devices with 'rapidly advancing AI' to enable the full creation of a smart system and reap the associated benefits [5].
Despite apprehension, it’s thought the manufacturing industry is where AI has the most impact [6]. As such, it’s strange that a large part of industry remains dubious about the adoption of the technology, especially considering the high stakes.
“The textile industry is a massive market with many inefficiencies,” says Gilberto Loureiro, co-founder at Smartex, a developer of computer vision systems for quality and control purposes. “Usually, it presents massive opportunities when technology improves part of the [manufacturing] process.”
3. Implementation
So, why is adoption still so slow?
Oyekan, of the University of Sheffield, says the slow adoption of AI may be down to the use of traditional systems. Oyekan works with small-to-medium-sized enterprises (SMEs) and larger manufacturers to examine areas that might benefit from AI technologies, and through his work noticed the struggle of getting legacy equipment hooked up to AI systems.
“Getting data from […] legacy equipment is a big challenge. […] That’s one of the reasons that AI in manufacturing has still quite a journey to go,” Oyekan says.
Microsoft’s Hall concurs by suggesting that the application of AI to manufacturing requires key foundational technologies to be in place – a factory must be networked to extract data from production lines [5]. This suggests that the implementation of AI first requires the adoption of smart factory technologies, a leap perhaps too big for facilities with traditional equipment.
But Oyekan draws attention to his work with the Advanced Manufacturing Research Centre (AMRC), a Sheffield, UK-based innovation facility. Here, researchers have examined how cheap, off-the-shelf sensors such as Microsoft Kinects could be applied to legacy machines to gather data. This implies solutions may be on the way from R&D teams.
Elsewhere, Oyekan says the infancy of the term Industry 4.0 and cash-wrapped SMEs has led to scepticism and apprehension around the commonly large investments into AI.
“Trying to adapt their processes so that AI can help them and work side-by-side with them is a challenge as well because of the cash investment they have to put in. I think […] that they have to see the benefits up front before they decide ‘okay, yes, we want to invest more into it’, and it’s very difficult for them to do because of them being cash-wrapped,” Oyekan explains.
Raj Koneru, founder and CEO at Anvizent, a company with the goal to operationalise AI, supports Oyekan’s claims: “AI uptake is rather slow among [small-to-medium-sized] manufacturers. While the large companies have begun to progress from plant automation to advanced analytics, many of the SME manufacturers haven’t even begun automating their plants.”
Koneru adds that the time-consuming nature of data management, along with implementation, accessibility and limitations to data storage, “continues to be among the biggest roadblock[s] to the adoption of AI”. Koneru predicts that this may change as AI- and cloud-based platforms launch with goals to operationalise AI through ‘pre-packaged use-cases’.
On the contrary, there are funding bodies instigating adoption among SMEs. The Made Smarter commission, a partnership between the UK government’s Department for Business, Energy & Industrial Strategy (BEIS) and industry leaders, launched a US$25.8m (GB£20m) programme to support SME manufacturers in the adoption of new, industrial digital technologies. Thus far, 300 north-west UK SMEs have secured support, with 62 of the businesses investing in AI, IoT and robotics to solve business challenges – among them, three textile firms: Panaz, Edward Taylor Textiles and Tibard [7].
However, this suggests monetary barriers can often only be smashed by investments. Thus, the adoption of AI on a global industrial scale seems to remain slow largely due to such cash and workload obstacles. But in the garment and apparel manufacturing industries, where investment into innovation is key to keeping up with trends like fast fashion and personalisation, implementation of AI could be perceived as somewhat faster.
Initially, the application of AI was not widely accepted in the labour-intensive clothing production industry, but the competitive global industry and need for low-cost production were said to be some of the main reasons for AI’s wider adoption [8, p. 131]. It’s likely because AI can be applied to all stages of garment production such as preproduction, production and postproduction operations [8, p. 117].
Thus, AI has changed the way some manufacturers think about and view their operations. Manufacturers have begun to see value in the technology for the betterment of processes and are invited to utilise new applications by wielding AI [3]. These applications fall into three categories: product; process; and insight applications [3]. It is in process and insight applications where the specific value-adding benefits to garment manufacturing can be found.
4. Garment manufacturing process applications
In planning and controlling the manufacturing journey from start-to-finish, companies often use enterprise resource planning (ERP) software. Phil Lewis, vice president, solution consulting, EMEA at Infor, an ERP cloud software provider, says: “AI is changing the fashion and wider manufacturing industry’s use of ERP, and is on the way to becoming prevalent.” Oyekan reiterates that the use of AI within manufacturing processes enables higher resource efficiency – machinery and assets can be monitored, communication improved, and better decisions can be made during operations.
Automated machinery and robotics are powered by AI to cater “to the increasing demand of consumers, reduce the number of faults and keep production cost low” [8, p. 123]. The use of AI and IoT technologies with automated machinery enables applications like the ‘intelligent manufacturing of clothing’ – eg, predictive quality control and the predictive maintenance of machinery.
“Data is collected from manufacturing processes,” Oyekan says. “Once the data is collected, the data from the input and output of that manufacturing system enables us to generate mathematical models for those manufacturing processes. And then, through the application of simulations and digital twin [technologies], we are able to actually play back all that data and use those models to highlight inefficiencies in any system. This enables us to start improving efficiency; [the technology provides] us with insights and knowledge on how to improve the manufacturing process.”
On a micro level, this means all assets and processes have a relationship with AI in some way so that monitoring can take place. For example, Anvizent’s Koneru says that AI can keep things running smoothly.
“AI can keep machines working more efficiently,” Koneru says, “by identifying patterns in sensor data and predicting when machines are in danger of breaking down. This allows for proactive asset maintenance, reducing repair costs and preventing downtime or accidents.”
These predictive abilities have enabled more rapid, refined and aware process management skills in both manufacturing processes and in spotting defects in the final product. US-based Smartex is able to automate quality control processes in the textile industry.
“The Smartex vision system behaves as a person would during textile inspection,” says co-founder Loureiro. “It stops the production in case of faults, alerts employees in case of changes in patterns and sometimes is even more accurate than a real human inspector.”
Loureiro claims this can reduce defective production in the textile industry from “up to ~5% to close to 0%”, which has attracted attention from global clientele and funding bodies including Fashion For Good. The Smartex system integrates smart vision devices that utilise AI into production machinery like circular knitting machines and more, enabling monetary and raw material savings through removing inefficiencies.
Oyekan notes this as one key benefit of the implementation of AI: “How do you inspect defects on the manufacturing line? In a manufacturing system that is producing a lot of materials – if you have an efficient system that is able to take rapid pictures of products as they come through manufacturing lines – you can start understanding why certain products have defects and why others don’t. Together with AI, you can start using data downstream or upstream in the manufacturing process to understand why those defects are happening.”
Similar abilities can even be used for identification. For example, while fibre identification traditionally involves using ‘destructive’ harsh chemicals, AI can act as a more convenient solution: the technology can identify and grade textile fibres according to colour, fineness, length, uniformity ratio, tenacity and “effect of spinning performance on yarn properties” [8, p. 117-118]. AI systems can even be used to determine the sewability of different fabrics [8, p. 124] and simplify the monitoring of the quality of the finished garment [9].
Virtual modelling, prediction of yarn tensile properties and prediction of yarn unevenness are but a few other benefits of implementing AI. However, the nature of the textile manufacturing industry means that there are often several operational variables, which means R&D is focused on knowing, analysing and generalising these yarn quality predictions [8, p. 118].
But predictive maintenance, quality control and identification represent only a few new processes enabled by AI.
AI also has applications for designers, for example. It’s seen in new, emergent computer-aided design (CAD) systems that can simulate 3D garment previews [8, p. 124]; in January 2020, Chinese sustainable fashion start-up HeartDub introduced an AI-based technology to its processes that can gather data on textile materials to create virtual fabric simulations, enabling production development costs to be cut down [10].
Elsewhere, in academic research, an interactive genetic algorithm (IGA) was developed that utilises AI to automatically create clothing patterns and predict optimal fashion designs based on human preferences and emotions [11]. The developments in AI technologies are enabling capabilities previously unseen and unimaginable in industry.
The garment assembly process is also becoming more automated in a bid to cater to increasing pressures from customers [8, p. 123] and fast fashion. This automation is enabled by AI. Spinning and weaving automation exists to ease textile manufacturing, while robotic sewing and cutting machines, robot and cobot assembly arms etc, handle garment manufacture. US-based Softwear Automation, for example, creates robots that can perform advanced sewing duties on textile and garment manufacturing lines.
Faughnan of EC Electronics says AI also helps automation systems communicate with each other and operators in real-time, enabling the efficient management of assets, inventory and supply chains. Known as machine-to-machine (M2M) communication, this allows for the streamlining of the production line and lets machinery automatically read and follow CAD instructions without the need for manual programming [12].
The use of AI-enabled automation technologies has also helped to relieve humans of difficult and dangerous duties in manufacturing spaces.
“On large-scale manufacturing activities, a lot of people get injured yearly,” Oyekan says. He continues, drawing attention to the potential for a more collaborative relationship between humans in automation in the future: “If you can get a robot that can work alongside the human to do [certain] tasks, the human is protected; the robot does the task, and then the human goes back onto that product and does all the intricate things that the robot can’t currently do.
“That’s my hope for the future – getting that balance of collaboration happening.”
The adoption of AI is therefore a decision of safety for some operations. On the other hand, AI may represent a move toward transparency and insight.
5. Garment manufacturing insight applications
AI enables an analytical glimpse into the manufacturing process, supply chain, retail sector and wider market intelligence by wielding data. Traditionally, acquiring data was performed manually, but AI has become a dependable tool that can connect the full manufacturing process and organise data on a company’s behalf.
Oyekan explains this has helped in terms of human efficiency: “What we’ve seen is that manufacturing efficiency improves because [these technologies] take away the human factor,” he says.
According to the digital manufacturing professor, this is especially important for ‘dull, boring and repetitive tasks’ because humans tend to get tired. Data acquisition is a lengthy process and thus is better suited to the abilities of AI. In the manufacturing space, companies have a ‘multitude of internal data sources’ too, says Anvizent CEO, Koneru.
“This includes their various operational systems, data from subsidiaries, data from shop floor systems, etc. Now, there’s an opportunity to ingest and process data from external or new sources such as commodity prices, currency changes, weather information, public information, and so on.
“The combination of this internal and external data has the potential to generate a whole new level of intelligent insights that generate high value. AI technology helps realise this potential; it takes analytics beyond explaining what has happened, to predicting what is likely to happen, and even suggesting the best course of action to achieve desired results.”
External data could also take shape from the supply chain itself – there are various AI approaches for supply chain planning [13], supply chain demand forecasting [14], optimisation of the supply chain network [15], managing logistics in the textile supply chain [16] and inventory management [17] and replenishment [8, p. 128, 18, 19].
AI insights can also be taken from areas of retailing. Insight into sales forecasting, fashion retail forecasting, style suggestion to consumers, customer relationship management (CRM), customer satisfaction and fashion coordination represent a handful of areas where AI application and data extraction is ‘ever increasing’ [8, p. 128].
AI helps here because, for example, “historical data on sales, in combination with style, colour and garment size, can be used for sales forecasting” [8, p. 128]. The analytical processing power of AI can even be used as a suggestion system for customers, enable improved services [8, p. 128] and automate sizing tools.
Another key development surrounding AI in fashion retail was explored in Vogue Business by Silicon Valley entrepreneur and investor Peter Diamandis. He suggests that AI could supercharge the shopping experience by powering augmented reality (AR) technologies [20].
And regarding the logistics of retailing, Lewis of Infor adds that the company conducted a case study that found AI, in conjunction with other processes, helped one client to reduce inward goods processing times ‘from 30 minutes to 10 seconds’.
Thus, as the technology improves, the garment manufacturing supply chain could become rapidly efficient from planning through to retail.
However, Oyekan adds that the ethics of such practice is of fundamental importance: “It’s important that all this data from humans and processes is used in an ethical and safe manner to benefit humans.”
6. Future applications of AI
AI literature reiterates that the application of AI has largely not received wide acceptance on the production floor and that although the use of automation is increasing, the application of AI is limited [8, p. 131]. This exemplifies the low rate of adoption in the garment manufacturing industry; researchers suggest the limited availability and high cost of textile-specific software may be the cause of this [8, p. 131].
Nevertheless, researchers predict that future R&D into AI for textile and garment manufacturing will focus on select key areas. These include, among others: (a) improving the method of data collection; (b) improving prediction capabilities; and (c) improving man-machine interaction [8, p. 130-131].
The latter is a key focus in academia and industry. The University of Sheffield’s Oyekan shared with WTiN his wishes for a more collaborative robot-machine work environment; elsewhere, Koneru of Anvizent says AI developers are striving for a state that combines humans and machines effectively. Once tackled, this may help to multiply the AI-based solutions on offer for textile and garment manufacturing.
Elsewhere, other projects exist to aid the adoption and development of AI: the High Value Manufacturing (HVM) Catapult – a government-funded UK body that has ties with academia like the AMRC in Sheffield – offers an environment where SMEs can experiment with digital technologies like AI, with experts at the ready to offer advice.
“[By utilising the HVM Catapult], the SMEs can tell them what the problems are; the SMEs can test these algorithms in a safe environment. […] Once the SMEs are quite happy with what they see, they can go out there and deploy [the technology] onto the production environment,” Oyekan explains.
With a handful of initiatives helping AI grow its use cases and capabilities, industry may see the commoditisation of AI – this may aid the quest to energise global manufacturing. This is backed by Koneru, who suggests that AI developments may lead “to a significant reduction in complexity and cost and accelerate the commoditisation of AI” in industry.
Furthermore, as progress in AI continues, it will evermore become an important tool for garment manufacturers to “[enhance] quality, [increase] production, [lower] operating costs and [exercise] in-house control over production” [8, p. 131]. Faughnan of EC electronics says he is certain that, going forward, “we can expect to see AI have a positive impact on operational processes and speed, as well as trust and dependability”.
Figure 1. System integration of new-generation intelligent manufacturing [1]
Meanwhile, Anvizent’s Koneru adds that manufacturers across industries could see fully connected AI environments that can predict cause-and-effect throughout the entire manufacturing chain.
The thought leadership embracing and promoting AI also imagines future manufacturing concepts. In the aforementioned ‘new-generation intelligent manufacturing’ model, researchers put forward a conceptual ‘giant system’ that consists of ‘three functional subsystems’ including intelligent products, intelligent production and intelligent services and also includes the industrial internet and intelligent manufacturing cloud. See Figure 1, taken from Toward New Generation Manufacturing [1, p. 17].
Nevertheless, the future potential for AI in manufacturing is vast; as the technology advances, the garment industry could anticipate a multitude of solutions on the way.
For now, though, AI in manufacturing is still in its infancy, says Oyekan. The processes required to apply AI are not fully developed and he suggests there’s a long journey to go in terms of R&D for bottleneck issues like implementation on legacy equipment.
Thus, AI for manufacturing is surrounded by a juxtaposed atmosphere of support, enthusiasm, reluctance, inaccessibility, promotion and continual forward-thinking development. Despite the obstacles, people are slowly waking up to the benefits it could offer businesses and the people they serve, Oyekan concludes.
-
References
[1] Ji, Z., Peigen, L., Yanhong, Z., Baicun, W., Jiyuan, Z. and Lui, M, “Toward New-Generation Intelligent Manufacturing,” in Engineering, 4, 2018, pp. 11-20
[2] SAS, “Artificial Intelligence – what it is and why it matters,” in SAS, n.d. [Online]. Available at: https://www.sas.com/en_gb/insights/analytics/what-is-artificial-intelligence.html [Accessed 3rd February 2020]
[3] Deloitte, “Artificial Intelligence Goes Mainstream,” in The Wall Street Journal, 2015. [Online]. Available at: https://deloitte.wsj.com/cio/2015/07/29/artificial-intelligence-goes-mainstream/ [Accessed 13th February 2020]
[4] Charalambous, E., Feldmann, R., Richter, G. and Schmitz, C., “AI in production: A game changer for manufacturers with heavy assets,” in McKinsey & Company, 2019. [Online]. Available at: https://www.mckinsey.com/business-functions/mckinsey-analytics/our-insights/ai-in-production-a-game-changer-for-manufacturers-with-heavy-assets [Accessed 6th January 2020]
[5] Hall, J., “The power of Artificial Intelligence in manufacturing,” in The Manufacturer, 2018. [Online]. Available at: https://www.themanufacturer.com/articles/power-artificial-intelligence-manufacturing/ [Accessed 13th February 2020]
[6] Kushmaro, P., “5 ways industrial AI is revolutionising manufacturing,” in CIO, 2018. [Online]. Available at: https://www.cio.com/article/3309058/5-ways-industrial-ai-is-revolutionizing-manufacturing.html [Accessed 28th February 2020]
[7] WTiN, “UK textile manufacturing SMEs adopt new technologies,” in World Textile Information Network (WTiN), 2020. [Online]. Available at: <https://www.wtin.com/article/f2f/2020/february/3_2_20/uk-textile-manufacturing-smes-adopt-new-technologies/> [Accessed 25th February 2020]
[8] Nayak, R. and Padhye, R., “Artificial intelligence and its application in the apparel industry,” in Automation in Garment Manufacturing, Elsevier, 2018, pp. 109-131
[9] Asia Textile Inspections Limited (ATI), “Role of Artificial Intelligence in Apparel Industry,” in ATI, 2018. [Online]. Available at: https://www.atihongkong.com/2018/08/role-of-artificial-intelligence-in-apparel-industry [Accessed 13th February 2020]
[10] Apparel Resources, “China start-up introduces AI-based technology for virtual fabric samples,” in Apparel Resources, 2020. [Online]. Available at: https://apparelresources.com/technology-news/manufacturing-tech/china-start-introduces-ai-based-technology-virtual-fabric-samples/ [Accessed 25th February 2020]
[11] Kim, H.-S. and Cho, S.-B., “Application of interactive genetic algorithm to fashion design,” in Engineering Applications of Artificial Intelligence, 13 (6), 2000, pp. 635-644
[12] Link, J., “Digitalising the traditional textile market,” in World Textile Information Network (WTiN), 2019. [Online]. Available at: https://www.wtin.com/article/2019/june/100619/digitalising-the-traditional-textile-market/ [Accessed 26th February 2020]
[13] Chiu, M. and Lin, G., “Collaborative supply chain planning using the artificial neural network approach,” in Journal of Manufacturing Technology Management, 15 (8), 2004, pp. 787-796
[14] Carbonneau, R., Laframboise, K. and Vahidov, R., “Application of machine learning techniques for supply chain demand forecasting,” in European Journal of Operational Research, 184 (3), 2008, pp. 1140-1154
[15] Yu, Y., Choi, T.-M. and Hui, C.-L., “An intelligent fast sales forecasting model for fashion products,” in Expert Systems with Applications, 38 (6), 2011, pp. 7373-7379
[16] Chandra, C. and Kumar, S., “An application of a system analysis methodology to manage logistics in a textile supply chain,” in Supply Chain Management: An International Journal, 5 (5), 2000
[17] Paul, S. and Azeem, A., “An artificial neural network model for optimisation of finished goods inventory,” in International Journal of Industrial Engineering Computations, 2 (2), 2011, pp. 431-438
[18] Dong, A. and Leung, S., “A simulation-based replenishment model for the textile industry,” in Textile Research Journal, 79 (13), 2009, pp. 1188-1201
[19] Pan, A., et al., “Optimal reorder decision-making in the agent-based apparel supply chain,” in Expert Systems with Applications, 36 (4), 2009, pp. 8571-8581
[20] McDowell, M., “A top Silicon Valley futurist on how AI, AR and VR will shape fashion’s future,” in Vogue Business, 2020. [Online]. Available at: https://www.voguebusiness.com/technology/ai-ar-and-vr-shaping-fashions-future-peter-diamandis [Accessed 26th February 2020]